Google’s DeepMind Reveals AI That Plays Games Just Like Humans
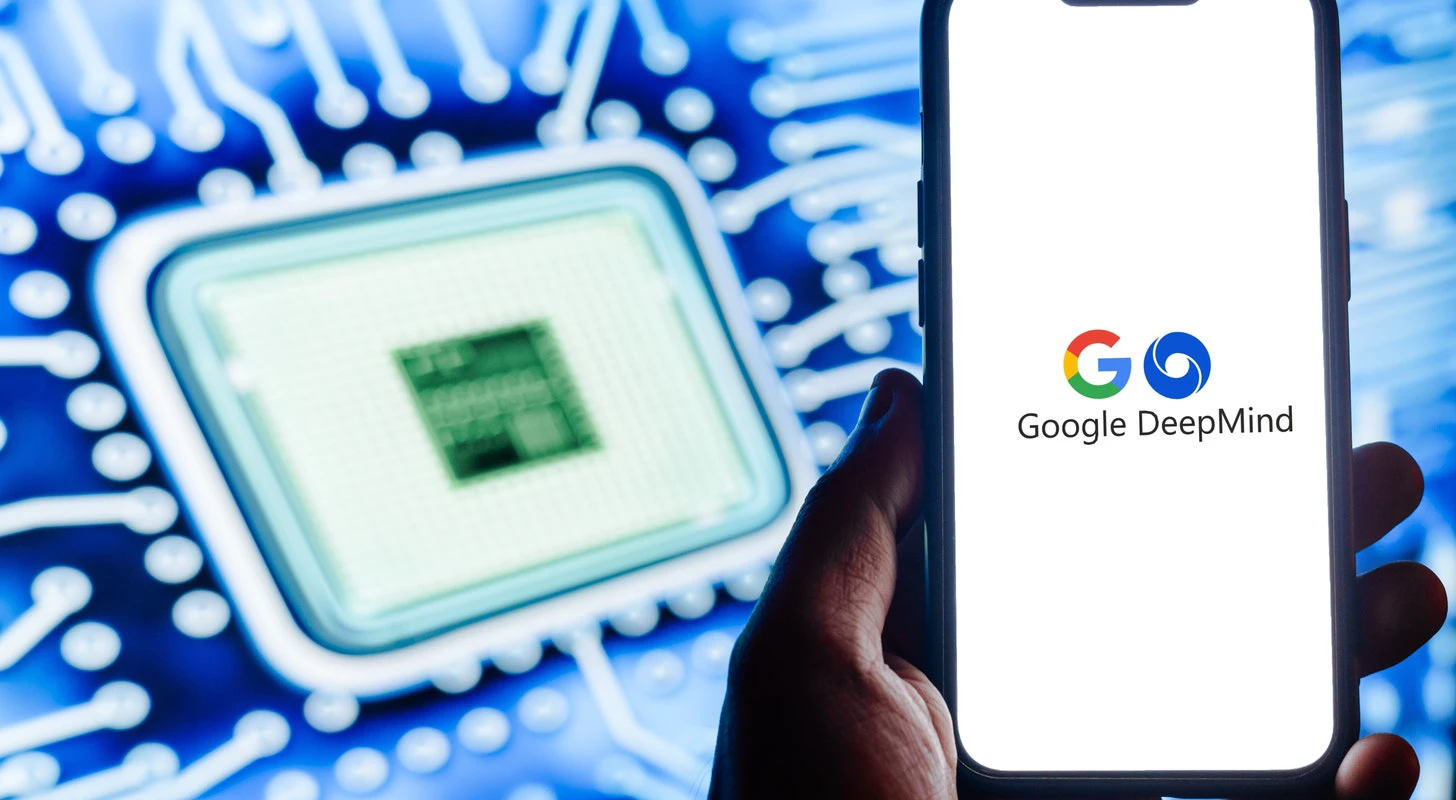
Alphabet Inc’s (NASDAQ: GOOGL) Google’s DeepMind has introduced the Scalable Instructable Multiworld Agent (SIMA), an artificial-intelligence (AI) model capable of playing various video games with human-like abilities.
Known for its capacity to understand natural language instructions and execute tasks across diverse virtual environments, SIMA represents a significant milestone in AI development.
“This research marks the first time an agent has demonstrated it can understand a broad range of gaming worlds, and follow natural-language instructions to carry out tasks within them, as a human might,” Google’s blog post reads.
By partnering with game developers and training on nine different games including No Man’s Sky and Valheim, SIMA showcases a remarkable ability to navigate complex scenarios and perform tasks such as shooting asteroids or driving a car autonomously.
Unlike traditional AI systems, SIMA doesn’t require access to game source codes or custom APIs, relying instead on simple inputs like on-screen images and natural language instructions.
It has been evaluated across 600 basic skills, demonstrating proficiency in navigation, object interaction, and menu use within a ten-second timeframe.
Furthermore, SIMA’s training across multiple games proves advantageous, as it outperforms specialized agents trained on individual games and exhibits the ability to generalize its skills to unfamiliar environments.
DeepMind’s research with SIMA not only advances AI capabilities in gaming but also lays the groundwork for more versatile and helpful AI agents in various real-world applications.
By continuing to refine SIMA’s abilities and incorporating more sophisticated models, DeepMind aims to develop AI systems capable of understanding and executing complex tasks, ultimately contributing to the creation of more generalized and beneficial AI technologies.